Artificial intelligence applied to coronary artery calcium scans (AI-CAC) significantly improves cardiovascular events prediction
- carl36250
- Nov 5, 2024
- 17 min read
Updated: Nov 11, 2024
https://www.nature.com/articles/s41746-024-01308-0 - Published: 05 November 2024.
Naghavi M, Reeves AP, Atlas K, Zhang C, Atlas T, Henschke CI, Yankelevitz DF, Budoff MJ, Li D, Roy SK, Nasir K, Molloi S, Fayad Z, McConnell MV, Kakadiaris I, Maron DJ, Narula J, Williams K, Shah PK, Levy D, Wong ND. Artificial intelligence applied to coronary artery calcium scans (AI-CAC) significantly improves cardiovascular events prediction. NPJ Digit Med. 2024 Nov 5;7(1):309. doi: 10.1038/s41746-024-01308-0. PMID: 39501071; PMCID: PMC11538462.
Abstract
Coronary artery calcium (CAC) scans contain valuable information beyond the Agatston Score which is currently reported for predicting coronary heart disease (CHD) only. We examined whether new artificial intelligence (AI) applied to CAC scans can predict non-CHD events, including heart failure, atrial fibrillation, and stroke. We applied AI-enabled automated cardiac chambers volumetry and calcified plaque characterization to CAC scans (AI-CAC) of 5830 asymptomatic individuals (52.2% women, age 61.7 ± 10.2 years) in the multi-ethnic study of atherosclerosis during 15 years of follow-up, 1773 CVD events accrued. The AUC at 1-, 5-, 10-, and 15-year follow-up for AI-CAC vs. Agatston score was (0.784 vs. 0.701), (0.771 vs. 0.709), (0.789 vs. 0.712) and (0.816 vs. 0.729) (p < 0.0001 for all), respectively. AI-CAC plaque characteristics, including number, location, density, plus number of vessels, significantly improved CHD prediction in the CAC 1–100 cohort vs. Agatston Score. AI-CAC significantly improved the Agatston score for predicting all CVD events.
Introduction
Coronary artery calcium (CAC) scoring is the strongest predictor of risk for atherosclerotic cardiovascular disease (ASCVD) in asymptomatic individuals1. Although CAC scoring is used for prediction of coronary heart disease events, it is not used for prediction of other cardiovascular disease (CVD) events such as stroke, heart failure (HF) and atrial fibrillation (AF). Beyond risk factor assessment, screening tools for overall CVD event prediction are limited due to cost-effectiveness and feasibility barriers.
The usage of CAC scans has increased significantly since the ACC/AHA Guideline on the Management of Blood Cholesterol in 20182 included CAC score in the algorithm for consideration of statin therapy, among those at borderline and intermediate risk for ASCVD. It is estimated that 45-50% of the US population aged 40-80 would fall in these groups defined as 5-20% risk of ASCVD events over 10 years3,4. The possibility of applying artificial intelligence (AI) to predict CVD has been previously published by some of our team members using the support vector machine algorithms in MESA5. We have sought to further enrich the value of CAC scans by applying AI that automatically measures all cardiac chamber volumes and left ventricular (LV) mass without using any contrast agent. For this manuscript, we refer to AI-enabled automated cardiac chambers volumetry from CAC scans as AI-CAC, and the AI-CAC model incorporates Agatston CAC Score, left atrial (LA), right ventricular (RV), left ventricular (LV) volume and mass.
We have recently shown that AI-CAC volumetry alone enabled the prediction of HF in the Multi-Ethnic Study of Atherosclerosis (MESA)6,7. Additionally, we have demonstrated that AI-CAC LA volume alone improved the predictive value of CHARGE-AF Risk Score and NT-proBNP for the detection of individuals at high risk of AF8,9. Such an add-on measurement can offer valuable insights into a patient’s overall CVD risk beyond the CAC score. In this study of MESA participants, we compared the performance of AI-CAC over the traditional Agatston CAC Score for the prediction of all CVD events (stroke, myocardial infarction, angina, resuscitated cardiac arrest, all cardiovascular disease-related deaths, HF, and AF) plus all-cause mortality. This approach broadens the scope and clinical significance of comparing AI-CAC vs. the Agatston CAC score.
Results
The mean (SD) age of our subjects was 62 ± 10 years, 52% were female, 40% were non-Hispanic White, 26% non-Hispanic Black, 22% Hispanic, and 12% Chinese. Table 1 shows the baseline characteristics of MESA participants who experienced a CVD event vs. those who did not over the 15 years of follow-up, during which 1773 CVD events accrued. In univariate comparisons, participants experiencing CVD events were older, more likely male, and more likely non-Hispanic White. The cases that experienced a CVD event had higher cardiac chamber volumes for LA, LV, RA, and LV mass.
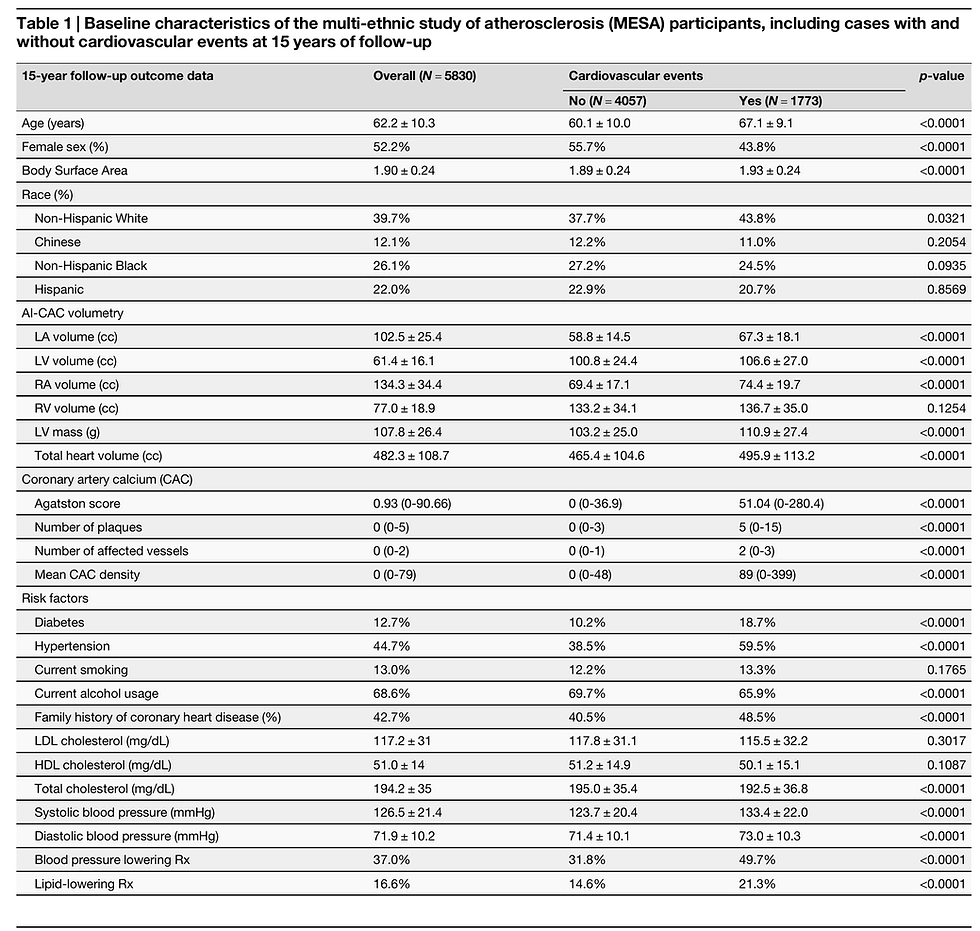
Figure 1 shows examples of three participants with enlarged LA and LV volumes with CAC score 0 and low risk ( < 5%) ASCVD risk score who experienced CVD events. A significant number of low-risk participants with CAC 0 have enlarged cardiac chambers. With a higher CAC score category, there was a higher proportion of patients with LA and LV volumes in the highest quartile (p-trend = 0.0001). 17.7% of cases with CAC 0 who are considered low risk have enlarged LA volume that puts them at high risk for AF and stroke (Fig. 2a). Similarly, 22.7% of cases with CAC 0 have enlarged LV volume that puts them at risk of HF (Fig. 2b).
Fig. 1: AI-enabled automated cardiac chambers volumetry and calcified plaque characterization to CAC scans (AI-CAC) definition and case examples.
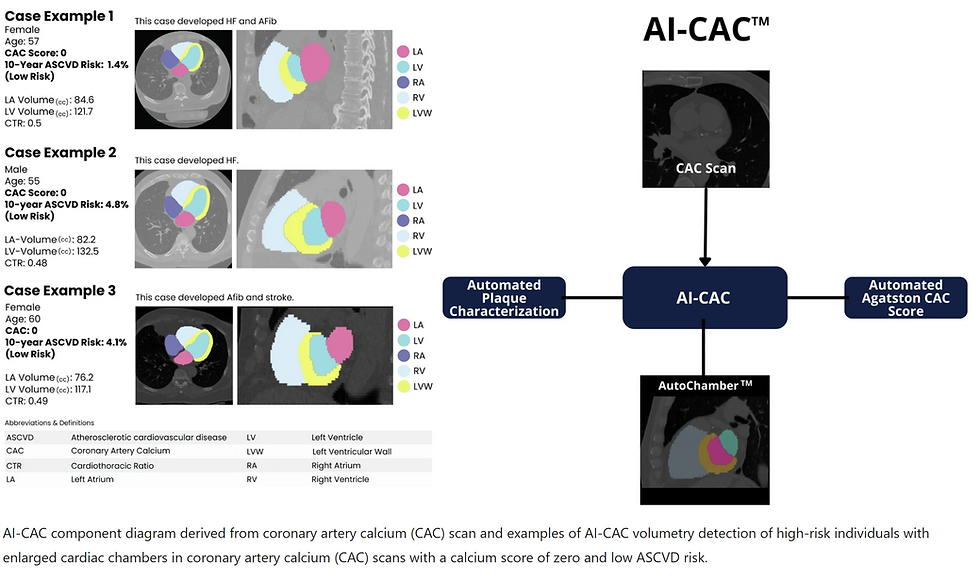
Fig. 2: Quartiles of AI-enabled automated cardiac chambers volumetry and calcified plaque characterization to CAC scans (AI-CAC) Left Atrial (LA) and Left ventricular (LV) Volume by Agatston Coronary Artery Calcium (CAC) Score Quartiles.
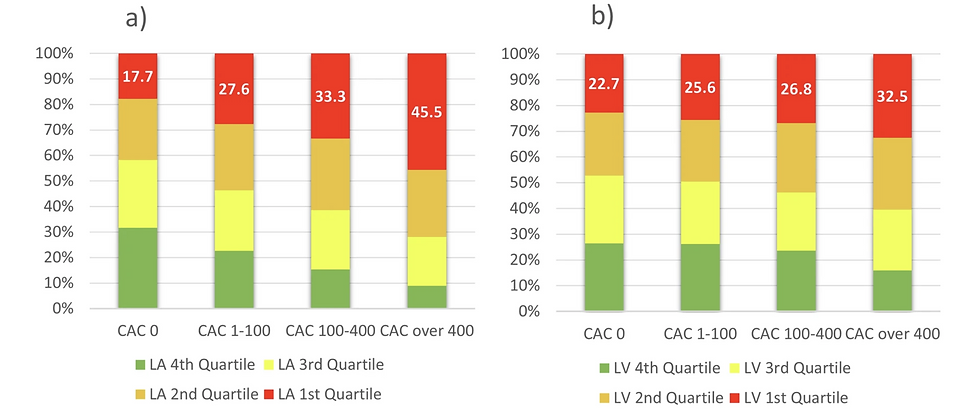
a AI-CAC LA volume vs. CAC score. Stacked bar chart of quartiles of AI-CAC LA volume by CAC score categories (0, 1–100, 101–400, over 400). Despite the correlation, 17.7% of cases with CAC 0 who are considered low risk have enlarged LA volume that puts them at high risk for atrial fibrillation (AF) and stroke.
b AICAC LV volume vs. CAC score. Stacked bar chart of quartiles of AI-CAC LV volume by CAC score categories (0, 1–100,101–400, over 400). 22.7% of cases with CAC 0 have enlarged LV volume that puts them at risk of heart failure (HF).
The median C-statistic (95% CI) for all CVD events over 15 years for pooled sexes between AI-CAC vs. Agatston CAC score was 0.742 (CI: 0.723–0.761) vs. 0.709 (CI: 0.688–0.728) (p < 0.0001). For females, the C-statistic between AI-CAC volumetry vs. Agatston CAC Score was 0.751 (0.738–0.778) vs. 0.705 (0.683–0.720) (p < 0.0001), respectively, and 0.701 (0.674-0.723) vs. 0.672 (0.651–0.693) (p = 0.0012), respectively, for males. AI-CAC had significantly higher discrimination than Agatston CAC Score for CVD events prediction across 1-, 5-, 10-, and 15-year follow-up (Fig. 3), including AF, HF, stroke, hard CVD, and All-cause mortality prediction (Table 2). Category-free NRI showed improvement across all follow-up periods for AF, HF, stroke, hard CVD, and All-Cause Mortality.
Fig. 3: Time-dependent receiver operating curve (ROC) area under curve (AUC) for all cardiovascular events between AI-enabled automated cardiac chambers volumetry and calcified plaque characterization to CAC scans (AI-CAC) vs. Agatston coronary artery calcium (CAC) Score over 15 years.

a Time-dependent AUC for AI-CAC vs. CAC score at 1-year follow-up. AI-CAC had significantly higher discrimination than Agatston CAC score for CVD events prediction over 1-year follow-up. The AUC at 1-year follow-up for AI-CAC vs. Agatston Score was 0.784 vs. 0.701 (p < 0.0001). b Time-dependent AUC for AICAC vs. CAC score over 5-years follow-up. At a 5-year follow-up, AI-CAC continued to demonstrate superior discrimination compared to the Agatston CAC score. The AUC for AI-CAC vs. Agatston score was 0.771 vs. 0.709 (p < 0.0001). c Time-dependent AUC for AI-CAC vs. CAC score over 10-years follow-up. For a 10-year follow-up, AI-CAC maintained a higher AUC compared to the Agatston score (0.789 vs. 0.712, p < 0.0001). d Time-dependent AUC for AI-CAC vs. CAC score over 15-years follow-up. At the 15-year follow-up, AI-CAC achieved the highest discrimination, with an AUC of 0.816 vs. 0.729 for the Agatston score (p < 0.0001).
Table 2 Time-dependent area under the curve (AUC) and category-free net reclassification index (NRI) at 1-, 5-, 10-, and 15-year follow-up between AI-CAC and Agatston CAC score (CAC) for individual and all cardiovascular event and mortality prediction
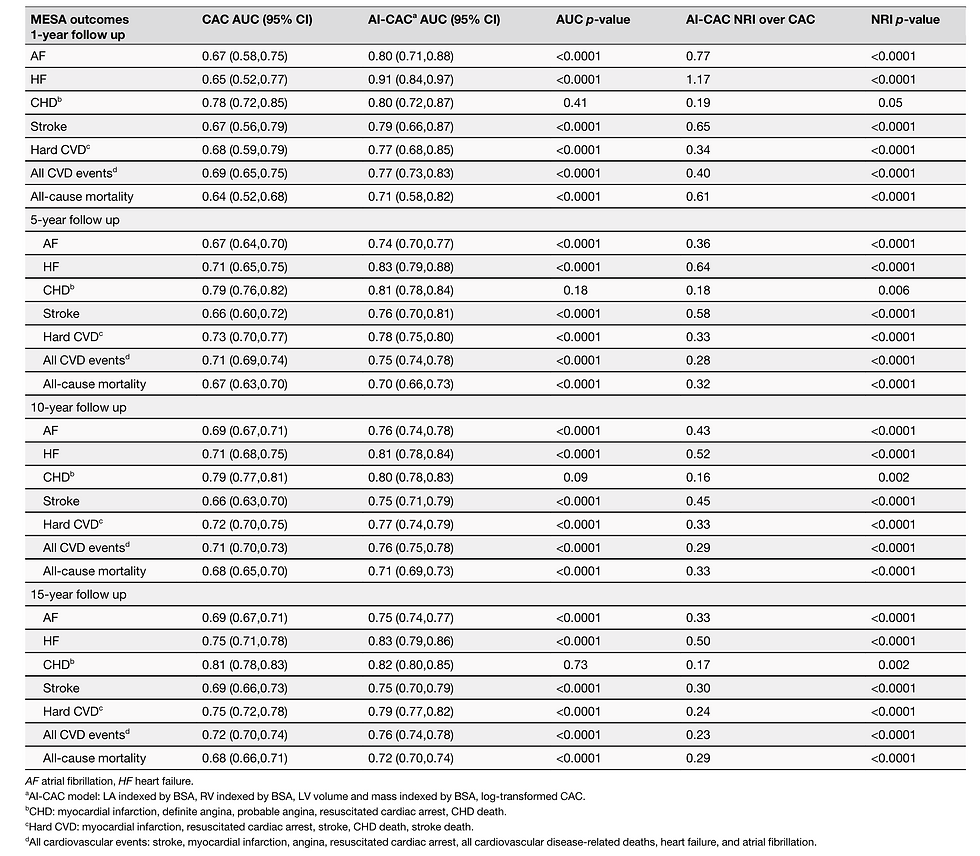
AI-CAC volumetry significantly added to a CVD risk factors-based model for all CVD event prediction (Supplementary Table 1). The AI-CAC biomarker model coefficients and hazard ratios for CVD, AF, and HF have been provided in Supplementary Tables 2, 3, 4. A significant increase in C-statistic for All CVD events was observed when adding AI-CAC measurements to basic risk factor models (0.745 (0.655–0.836) vs. 0.774 (0.693–0.852)) (Supplementary Table 5). A notable increase in discrimination for CVD prediction was demonstrated over 1-, 5-, 10-, and 15-year follow-ups. AI-CAC measurements demonstrated significant incremental value when added to CVD risk factors for 1-year CVD prediction (0.803 vs. 0.749, p < 0.0001) (Supplementary Fig. 6a), 5-year CVD prediction (0.786 vs. 0.752, p < 0.0001) (Supplementary Fig. 6b), 10-year CVD prediction (0.801 vs. 0.774), p < 0.0001 (Supplementary Fig. 6c), and 15-year CVD prediction (0.823 vs. 0.816, p < 0.0001) (Supplementary Fig. 6d).
AI-CAC plaque characterization significantly improved CHD prediction in the CAC 1–100 cohort. AI-CAC plaque characteristics included the number of plaques, location, density, plus number of vessels affected. The addition of AI-CAC RV volume, LV volume, and LV mass further improved discrimination for CHD in this cohort. The AI-CAC composite model included LA volume, RV volume, LV volume, LV mass, AI-CAC derived plaque characterization, and Agatston CAC Score. Over 5- and 10-year follow-up, the time-dependent AUC for the AI-CAC composite model vs. Agatston CAC Score was 0.654 vs. 0.557 (p < 0.0001) and 0.688 vs. 0.556 (p < 0.0001), respectively (Supplementary Fig. 8a, b).
Discussion
Our study primarily demonstrates the utility of applying AI to CAC scans to extract more actionable information than currently reported, which is the Agatston CAC score only. We found that AI volumetry significantly improves upon traditional CAC scoring for the prediction of risk for total CVD events as well as the prediction of individual CVD events of HF, stroke, AF, and all-cause mortality in a large multi-ethnic cohort. The plaque characterization component of AI-CAC specifically improved the predictive value of the Agatston score for CAC scores 1–100. Moreover, we show the value of this technique not only for longer-term event prediction (10-15 years) but also for nearer-term events (1 to 5-year follow-up). This is the first multi-ethnic outcome study of an easily implemented AI technology that can be applied to non-contrast CAC scans without additional radiation exposure to identify patients at risk of such events who would otherwise not be identified by Agatston CAC score. The potential utility of non-coronary findings in CAC scans has been reported previously using manual 2D measurements of LV10,11,12,13 and LA sizes14,15,16,17. Our study corroborates findings from the Heinz Nixdorf Recall Study and others and further brings to light the value of non-coronary findings in CAC scans for a comprehensive CVD risk assessment beyond CHD14,15,16,17,18. Kizer et al. showed that LA size was an independent predictor of CVD events19. Mahabadi et al.15 showed in the longitudinal Heinz Nixdorf Recall Study that two-dimensional LA size and epicardial adipose tissue from non-contrast CT were strongly associated with prevalent and incident AF and that LA size diminished the link of epicardial adipose tissue with AF, and was also associated with incident major CVD events independent of risk factors and CAC-score16.
Although there are multiple automated CAC scoring tools available, currently, there is no clinically available tool to clinicians for automated cardiac chamber volumetry in CAC scans that is validated against outcomes. Here, we provide evidence of the feasibility of using AI for automated 3D volumetry of cardiac chambers that takes on average 20 s. Currently, such measurements are only possible on contrast-enhanced CT scans, which require more radiation plus injection of an X-ray contrast agent that is burdensome20. In contrast, AI-CAC volumetry can be applied to any new or existing non-contrast CAC scan for automated cardiac chamber measurement. Standalone cardiac MRI and echocardiography are not comparable to our solution, which is an opportunistic add-on to chest CT scans. While echocardiography and cardiac MRI provide valuable information on cardiac chamber volume, they are not indicated for the asymptomatic population and are usually performed in cardiovascular clinics. However, AI-enabled cardiac chamber volumetry can be done on any chest CT scan, including lung cancer screening scans. This approach opens the door to identifying high-risk asymptomatic patients in non-cardiovascular clinics.
AI-CAC volumetry not only works on ECG-gated CAC scans but also non-gated lung CT scans21. Non-contrast chest CT scans are prime candidates for opportunistic AI-enabled cardiac chamber volumetry for the identification of patients at increased risk for AF22 and HF. The AI approach can enable automatic screening of the over 10 million chest CT scans done each year in the US alone23. Such an AI tool can run in the background of radiology picture archiving and communication systems (PACS) and alert providers to cases with enlarged cardiac chambers. Unfortunately, many high-risk patients with enlarged cardiac chambers are currently undetected and, therefore, untreated. Early detection of these cases can allow for close monitoring of progression to AF for stroke prevention and guideline-directed medical therapy for HF prevention. In our study, we have found the unadjusted correlations between Agatston CAC score and LA and LV volumes to be low (R = 0.20 and R = 0.10, respectively) (Supplementary Figs. 1 and 2), hence a substantial portion of the population with enlarged LA and LV chambers are found in low-risk CAC categories. The combination of the automated cardiac chambers volumetry component of AI-CAC plus automated AI-CAC plaque characterization showed a greater incremental AUC value over the Agatston score vs. each alone.
Finally, the lack of coverage for CAC scans by Medicare and healthcare insurance carriers has contributed to healthcare inequity in the US. Ikram and Williams24 have shown that low-income people in the Chicago area are less likely to get CAC scans compared to people in higher-income zip codes. We hope that by applying AI to CAC scans and providing incremental value, the payers will be more likely to cover CAC scans.
Our study has several strengths and limitations. The multi-ethnic nature of MESA recruited from six field centers around the US provides for greater generalization of our findings than single-center studies. MESA included standardized methods of data collection, laboratory measurement, follow-up, as well as adjudication of CVD events. Internal validation was not performed with hold-out due to the low sample size of several events.
One limitation is that the MESA Exam 1 baseline CT scans were performed between 2000 and 2002 using electron-beam computed tomography (EBCT) or earlier generation multidetector CT scanners, and current CAC scanning utilizes more advanced multidetector CT scanning. However, since our AI training was done completely outside of MESA and used a modern multidetector (256 slices) scanner, we do not anticipate this to affect the generalizability of our findings.
Another limitation is the potential impact of different ECG gating methods (RR-interval) used in MESA for multidetector CT (50%) and EBCT (80%). This discrepancy resulted in significant differences in LA volume between participants scanned with EBCT vs. MDCT (57.4 cc vs. 65.4 cc, respectively, p < 0.0001). However, LV volume, LV mass, and RV volume measurements were not affected by scanner type (Supplementary Table 7). The cumulative incidence of AF between LA volumes measured by different scanners showed similar results (Supplementary Figs. 10 and 11). Although interaction terms between LA volume and scanner type were tested and found to be non-significant for outcome prediction, questions remain on the extent of the impact of scanner type on our findings.
Since MESA does not distinguish between HF subtypes (heart failure with reduced ejection fraction (HFrEF) vs. heart failure with preserved ejection fraction (HFpEF)), we were unable to compare the prediction of HF subtypes. However, in a preliminary study, we obtained data from 75 patients who underwent both a cardiac CT scan and echocardiography at Harbor UCLA medical center25. AI-CAC LV volume index (LVVI) defined as LV volume divided by BSA was able to distinguish HFrEF vs. HFpEF comparably to echocardiography LVVI (Supplementary Fig. 9).
Finally, our study excluded 771 cases who did not consent to the use of their data by commercial entities. However, the baseline characteristics of these cases did not differ systematically with respect to the remaining participants, and we do not anticipate this to affect our findings.
In this study, we presented AI-CAC data on cardiac chambers volumetry and calcified plaque characterization obtained from existing CAC scans in a large multi-ethnic prospective study and compared it to Agatston CAC Score alone for prediction of all cardiovascular events (stroke, myocardial infarction, angina, resuscitated cardiac arrest, all CVD-related deaths, HF, and AF), over 15 years. AI-CAC significantly improved upon the Agatston CAC score for all cardiovascular events prediction (including all CHD in CAC 1–100 cohort), as well as total mortality. Moreover, significant improvement in risk prediction and reclassification of events was not only seen for longer-term (e.g., 10- and 15-year) events but also for nearer-term (e.g., 1- and 5-year) events, advancing the status quo to help identify individuals at risk of near-term CVD events and death.
The projected impact of our study lies in AI’s ability to provide opportunistic screening of enlarged cardiac chambers in all types of chest CT scans, including lung cancer screening and non-cardiac thoracic diagnostic CT scans. This manuscript primarily demonstrates the utility of applying AI to CAC scans to extract more actionable information than the Agatston CAC score that is currently reported. Additionally, AI-CAC can measure chamber volume much faster and cheaper and is operator-independent compared to manual methods.
Methods
Study population
MESA is a prospective, population-based, observational cohort study of 6814 men and women without clinical CVD at the time of recruitment. Six field centers in the United States participated in the study: Baltimore, Maryland; Los Angeles, California; Chicago, Illinois; Forsyth County, North Carolina; New York City, New York; and St. Paul, Minnesota. As part of the initial evaluation (2000-2002), participants received a comprehensive medical history, clinic examination, and laboratory tests. Demographic information, medical history, and medication use at baseline were obtained by self-report. An ECG-gated non-contrast CT was performed at the baseline examination to measure CAC (see below).
Outcomes
The primary outcome was a composite of all CVD events comprised of stroke, myocardial infarction, angina, HF, AF, resuscitated cardiac arrest, and all CVD-related deaths. Participants were contacted by telephone every 9–12 months during follow-up and asked to report all new CVD diagnoses. International Classification of Disease (ICD) codes were obtained. For participant reports of HF, coronary heart disease, stroke, and CVD mortality, detailed medical records were obtained, and diagnoses were adjudicated by the MESA Morbidity and Mortality Committee. Incident AF was identified by ICD codes 427.3x (version 9) or I48.x (version 10) from inpatient stays and, for participants enrolled in fee-for-service Medicare, from Medicare claims for outpatient and provider services. Hard CVD was defined as myocardial infarction, resuscitated cardiac arrest, stroke, CHD death, and stroke death. Angina was classified, except in the setting of MI, as definite, probable, or absent. Definite or probable angina requires symptoms of typical chest pain or atypical symptoms. Probable angina requires, in addition to symptoms, a physician's diagnosis of angina, and medical treatment for it. Definite angina required one or more additional criteria, including CABG surgery or other revascularization procedures; 70% or greater obstruction on coronary angiography; or evidence of ischemia by stress tests or by resting ECG. A detailed study design for MESA has been published elsewhere26. MESA participants have been followed since the year 2000. Incident AF has been identified through December 2018. 70 cases with AF diagnosed prior to MESA enrollment were removed from the analysis.
From the 6814 MESA participants, we excluded 771 who did not consent to the use of their data by commercial entities, leaving 6043 participants at baseline. Among the remaining participants, 125 participants with missing slices in CAC scans and 88 participants with missing event or time follow-up data were excluded, resulting in data from 5830 participants for final analysis. Of the 125 cases with missing slices in CAC scans were, 49.8% male and 50.2% female, with age 60.8 ± 10.1. These errors were random, and our investigations did not reveal any association between cases with missing slices and any of the dependent or independent variables in our study.
The AI tool for automated cardiac chamber volumetry
The automated cardiac chambers volumetry tool in AI-CAC referred to in this study is called AutoChamberTM (HeartLung.AI, Houston, TX). The deep learning model used TotalSegmentator27 as the base input and was further developed to segment each of the four cardiac chambers: LA, LV, RA, RV also in addition to several other components such as automated CAC score and plaque characterization, which are not presented here (Fig. 1). The core machine learning component of AI-CAC is adapted from TotalSegmentator which is a widely used anatomical model published and validated by investigators independent from our group. The source code for the TotalSegmentator base model is available publicly. The base architecture of the TotalSegmentator model was trained on 1139 whole-body CT cases with 447 cases of coronary CT angiography (CCTA) independent from MESA using nnU-Net, a self-configuring method for deep learning-based biomedical image segmentation28. The initial input training data were matched to non-contrast and contrast-enhanced ECG-gated cardiac CT scans with 1.5 mm slice thickness. Because the images were taken from the same patients in the same session, registration was done with good alignment. Following this transfer of segmentations, a nnU-Net deep learning tool was used for training the model. Additionally, iterative training was implemented whereby human supervisors corrected errors made by the model, and the corrected data were used to further train the model, leading to improved accuracy. To standardize the comparison in MESA, cardiac chambers were indexed by body surface area (BSA).
We developed a post-processing pipeline to identify instances of poor-quality segmentations where the region of interest was absent. Furthermore, we employed connected components analysis to eliminate ectopic segmentation islands. These methods were implemented to ensure quality control and optimize the model’s performance. Expert rules built into the AI model excluded 125 cases due to missing slices in image reconstruction, which occurred with some of the electron-beam CT scanners used in MESA at baseline.
Agatston CAC score measurement
Three study sites used cardiac-gated electron-beam CT scanners, whereas the other three sites used multidetector CT scanners. Each participant was scanned twice at baseline examination, with a mean Agatston score used for analysis29. All scans were phantom-adjusted and read by two trained CT image analysts at a central MESA CT reading center, with high reproducibility and comparability between electron-beam CT and multidetector CT scanning30,31. Detailed information on CT scan methods and interpretation has been provided previously30.
CAC area and density were derived from total Agatston and volume scores, which were provided in the original MESA data set. The methods for this derivation are elsewhere32.
AI-CAC plaque characterization beyond Agatston CAC score
In addition to AI-CAC cardiac chamber volumetry, AI-CAC enables calcified plaque characterization that currently is not reported by the Agatston CAC Score. These characteristics include the number of plaques, the number of vessels with plaques, plaque density, and location. In this study, we have only used these characteristics for calcified plaques, however, efforts are underway to characterize non-calcified (soft plaques) in non-contrast CAC scans using AI-CAC.
In MESA-1, human experts generated reports on plaque characteristics for each patient. For these reports, each expert manually identified plaques by clicking on them, extracting x, y, and z coordinates for each point, and measuring surface area using a connected components algorithm. This algorithm identified connected pixels that were adjacent side-by-side but excluded those connected diagonally. From these reports, we were able to extract information on plaque location, number of plaques, plaque density, and the number of vessels affected by plaques. We have chosen to use MESA’s plaque characterization due to excessive noise in the coronary arteries in MESA-1 CT scans.
Statistical analysis
We used SAS (SAS Institute Inc., Cary, NC) and Python 3.10 for statistical analyses. All values are reported as means ± SD except for CAC and plaque characteristics, which did not show normal distribution and are presented as median with interquartile range (IQR). All tests of significance were two-tailed, and significance was defined at Type I error (α) = 0.05 and Type II error (β) = 0.20. All analyses met the appropriate sample size and power considerations. Instances where these requirements were not met have been excluded and noted.
Survival analysis was performed using Cox proportional hazards regression. Model assumptions were tested using Schoenfeld and Martingale residuals and no violations of proportional hazards or non-linearity were detected in any variables. Discrimination was assessed using the time-dependent receiver operator characteristic (ROC) area under the curve (AUC)33 and Uno’s C-statistic34. The time-dependent AUC was calculated using the inverse probability of censoring weighting (IPCW) estimator without competing risks to determine discrimination at specific follow-up times. AUC confidence intervals were obtained using 1000 bootstrapped samples. Significance in the AUC difference between predictors was calculated based on the variance of the difference using the independent and identically distributed (iid)-representation of the AUC estimator. Uno’s C-statistic was calculated to account for significant right censoring over 15 years of follow-up for all CVD predictions (70%). Significance in concordance discrimination was determined using 1000 bootstrapped samples.
Category-free (continuous) net reclassification index (NRI) was calculated using the sum of the differences between the proportions of upward reclassifications and downward reclassifications events and non-events, respectively. P(up|event) and P(down|nonevent) form the positive components of the NRI in expression, while events that move down and non-events that move up are mistakes introduced by the new marker. NRI was developed as a statistical measure to evaluate the improvement in risk prediction models when additional variables are incorporated into a base model35.
The AI-CAC model, as presented, is comprised of the LA volume index, RV volume index, LV volume index, LV mass index, plaque characterization, and MESA-reported phantom-adjusted Agatston CAC score. Cardiac chamber volumetry was indexed by body surface area to standardize measurements. Because MESA participants were entirely asymptomatic without overt HF, LV volume and LV mass index demonstrated high collinearity and were combined into a composite variable. Correlation and variance inflation factor analysis showed low multicollinearity among the remaining predictors. Agatston CAC score was natural logarithm-transformed (ln-transformed + 1) to improve the interpretability of hazard ratios and avoid undue influence of large values. All predictors were modeled continuously and exhibited a linear relationship with outcomes.
The focus of this manuscript is comparing AI-CAC over Agatston CAC Score alone; therefore, no risk factors or other covariates were included in either model presented in the figures of this manuscript. However, incremental value of AI-CAC measurements over CVD risk factors have been provided in the Supplementary Information.
Comments